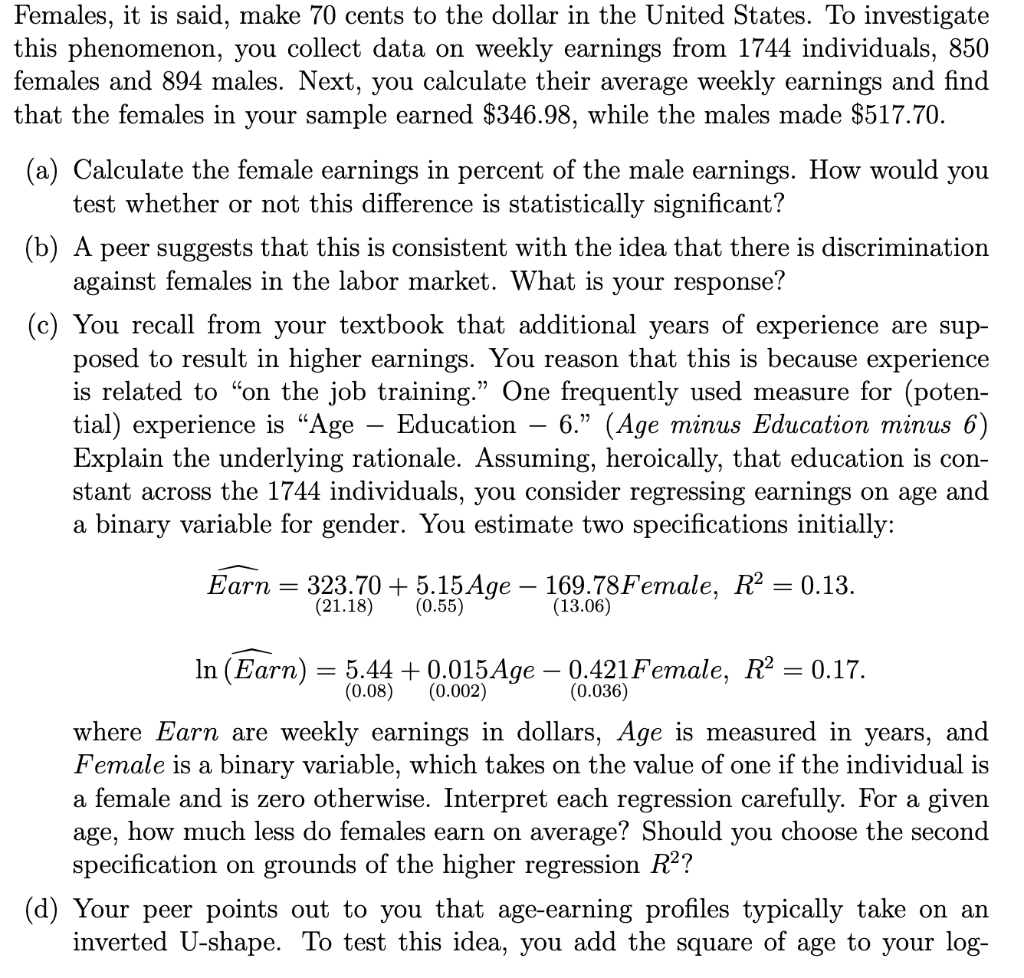
???????
Females, it is said, make 70 cents to the dollar in the United States. To investigate his phenomenon, you collect data on weekly earnings from 1744 individuals, 850 emales and 894 males. Next, you calculate their average weekly earnings and find that the females in your sample earned \( \$ 346.98 \), while the males made \( \$ 517.70 \). (a) Calculate the female earnings in percent of the male earnings. How would you test whether or not this difference is statistically significant? (b) A peer suggests that this is consistent with the idea that there is discrimination against females in the labor market. What is your response? (c) You recall from your textbook that additional years of experience are supposed to result in higher earnings. You reason that this is because experience is related to "on the job training." One frequently used measure for (potential) experience is "Age - Education - 6." (Age minus Education minus 6) Explain the underlying rationale. Assuming, heroically, that education is constant across the 1744 individuals, you consider regressing earnings on age and a binary variable for gender. You estimate two specifications initially: \[ \begin{array}{c} \widehat{\text { Earn }}=\underset{(21.18)}{323.70}+\underset{(0.55)}{5.15 \text { Age }}-\underset{(13.06)}{169.78 \mathrm{Female},} R^{2}=0.13 . \\ \ln \widehat{(\text { Earn }})=\underset{(0.08)}{5.44}+\underset{(0.002)}{0.015 \mathrm{Age}}-\underset{(.036)}{0.421 \mathrm{Female},} R^{2}=0.17 . \end{array} \] where Earn are weekly earnings in dollars, Age is measured in years, and Female is a binary variable, which takes on the value of one if the individual is a female and is zero otherwise. Interpret each regression carefully. For a given age, how much less do females earn on average? Should you choose the second specification on grounds of the higher regression \( R^{2} \) ? (d) Your peer points out to you that age-earning profiles typically take on an inverted U-shape. To test this idea, you add the square of age to your \( \log \) -
linear regression. \[ \ln (\widehat{\text { Earn }})=\underset{(0.18)}{3.04}+\underset{(0.009)}{0.147 \mathrm{Age}}-\underset{(0.033)}{0.421 \mathrm{Female}}-\underset{(0.0001)}{0.0016 \mathrm{Age}^{2}}, R^{2}=0.28 . \] Interpret the results again. Are there strong reasons to assume that this specification is superior to the previous one? Why is the increase of the Age coefficient so large relative to its value in (c)? What other factors may play a role in earnings determination?